- Introduction
- How AI is Transforming Recycling
- The Role of Machine Learning in Waste Segmentation
- Benefits of AI-Driven Recycling
- Challenges and Limitations
- Conclusion
- FAQs
- References
Introduction
As the world strives to reduce waste and improve sustainability, traditional recycling methods face significant challenges. Thankfully, recent technological advancements—particularly in artificial intelligence (AI)—are transforming the recycling industry. From improving waste segregation to increasing efficiency, AI-driven recycling offers a smart solution for environmental sustainability.
In this article, we will explore how AI is revolutionizing recycling methods, the role of machine learning in waste management, the benefits and financial impact of AI-driven recycling, and the associated challenges the industry continues to face. Let’s dive into each of these areas to understand the future of recycling through the lens of AI.
How AI is Transforming Recycling
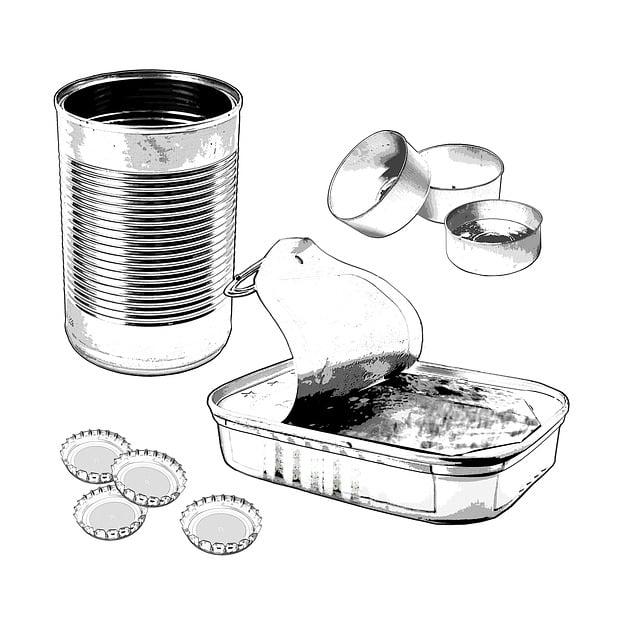
(Image: Pixabay/@Pumlan)
With the increasing complexity of waste streams, traditional recycling methods often fall short in effectively segregating mixed materials. AI is quickly becoming the go-to solution in addressing these inefficiencies. By using advanced sensors, computer vision cameras, and robotic arms, AI-powered machines can now efficiently recognize and sort different types of recyclables, from plastics and metals to glass and paper.
One standout innovation in the AI recycling space is the integration of neural networks that allow machines to "learn" about the waste they’re dealing with. These AI systems can identify various waste materials through object recognition software, ensuring every type of recyclable material goes into its correct waste stream.
Another way AI is transforming recycling is through real-time data. Smart sensors track and analyze the waste management process, helping companies and governments make data-driven decisions about where and how to optimize waste collection and processing. Brands like startup AMP Robotics are developing new technologies that can accelerate these processes dramatically.
Moreover, AI is also helping predict trends in consumer waste generation. By gathering historical and real-time data, AI algorithms generate insights about which materials are likely to be available for recycling at specific times, allowing cities to plan smarter waste collection services.
The Role of Machine Learning in Waste Segmentation
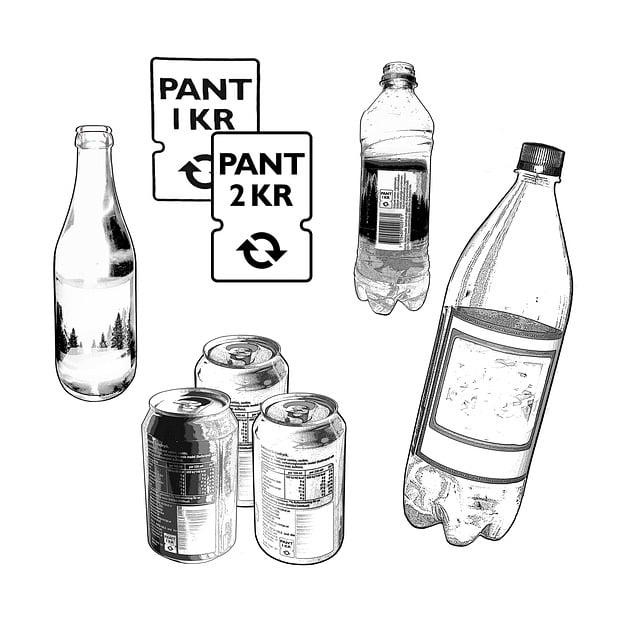
(Image: Pixabay/@Pumlan)
Much of AI-driven recycling depends on machine learning algorithms that enable continuous improvement over time. Machine learning models are constantly fed with data from recycling operations and work to refine their accuracy in classifying recyclable materials. The more data these AI systems process, the more they "learn," which results in better detection and handling of various waste items.
Machine learning enhances existing waste segmentation by automating tasks usually performed by human operators. Machine vision technology helps accurately distinguish between different types of plastics, such as PET (polyethylene terephthalate) and HDPE (high-density polyethylene), something that would take humans longer to accomplish manually. Not only does this reduce errors, but it also speeds up the overall process.
Additionally, as the technology evolves, machine learning algorithms become self-regulating, meaning they can adjust based on changing waste compositions. For example, if new packaging materials enter the market, a smart recycling system will eventually be able to classify and sort them after being exposed to enough instances of the new material.
Such developments are crucial for industries that produce reusable materials, as high-accuracy sorting leads directly to the reuse of quality resources instead of filling up landfills. AI-enabled waste segmentation becomes especially important in the current era of widespread efforts to curb plastic waste.
Benefits of AI-Driven Recycling
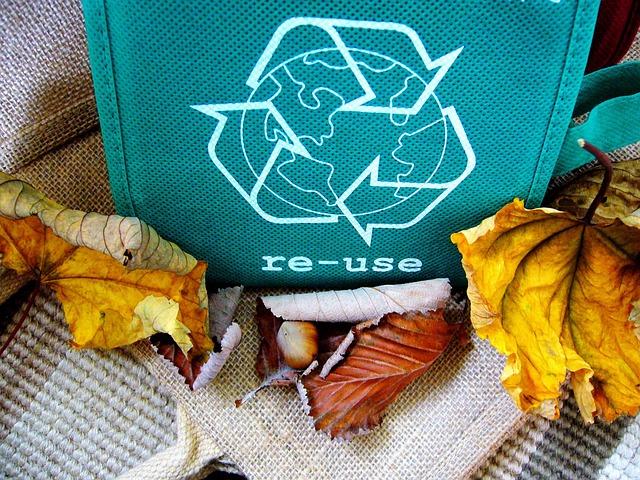
(Image: Pixabay/@Shirley810)
There are several tangible benefits associated with AI-driven recycling, ranging from improved operational efficiency to reduced environmental impact. Below are some of the major advantages:
1. Increased Efficiency: AI-based systems are fast, minimize downtime, and can dramatically increase throughput. Automated waste sorting powered by robots works around the clock and at a faster pace compared to human workers.
2. Cost-Effectiveness: While the initial setup cost of AI recycling technologies may seem high, these systems pay off long-term by reducing manpower, improving sorting accuracy, and minimizing processing errors. Moreover, consistent improvements from machine learning translate into better resource allocation and additional savings.
3. Environmental Impact: With the use of AI, recycling facilities can cut down on the volume of recyclable materials that cross into landfills, leading to measurable improvements in sustainability efforts. AI increases recycling rates, decreases contamination in recycled materials, and makes the overall process greener.
4. Predictive Capabilities: AI used for data analytics allows waste management companies to forecast the availability of materials, contributing to smarter logistics and route management. Predictive modeling also supports strategic investment decisions by identifying future material recovery trends.
Challenges and Limitations
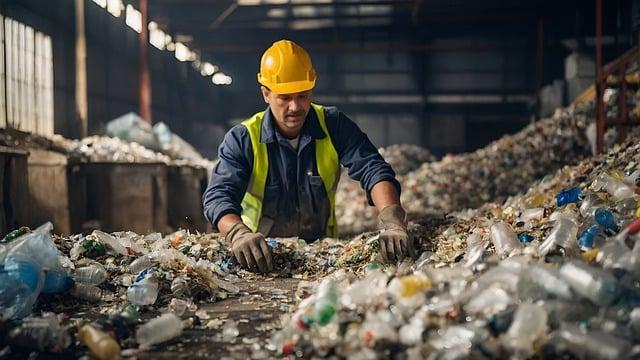
(Image: Pixabay/@geralt)
While AI presents many opportunities for advancing recycling, there are challenges that currently limit its full adoption across the globe. A few key hurdles are:
1. High Capital Costs: AI-powered solutions, including robotic arms and sophisticated sensors, require substantial upfront investments that may not be accessible to all municipalities or companies. Smaller recycling firms may struggle to afford the shift to AI automation.
2. Technology Integration: Current recycling plants often rely on legacy infrastructure. Retroactively integrating AI systems into outdated machinery can be a complex, and sometimes nearly impossible, project that requires coordinated adaptation and retraining.
3. Evolving Waste Complexities: Although AI can manage current waste complexities, it still lags when new and unpredictable waste forms emerge. Continuous upgrades and recalibration of AI systems may be required as recycling needs evolve.
4. Job Displacement: Like many automation technologies, AI-driven recycling could significantly reduce the demand for human labor in certain roles, which has raised concerns regarding job losses within the waste management sector. Finding a balance between automation and employment will be critical.
Conclusion
AI-driven recycling represents a step change in the global effort toward sustainable waste management. By enhancing sorting capabilities, modernizing waste segmentation techniques, and offering predictive analytics, AI is making recycling operations smarter and more environmentally friendly. While obstacles such as high investment costs and challenges in technology integration exist, the potential benefits suggest AI will play a crucial role in the advancement of the recycling industry for years to come.
FAQs
What is AI-driven recycling?
AI-driven recycling refers to the integration of artifical intelligence—such as machine learning algorithms and robotics—into the recycling process to improve efficiency, accuracy, and environmental outcomes.
How does AI help with waste sorting?
AI systems use advanced sensors, computer vision, and robotics to identify and separate different materials based on texture, size, and type, ensuring highly accurate sorting compared to traditional methods.
Can AI make recycling cheaper?
Yes. While its adoption involves an initial investment, AI-driven systems save money in the long run through enhanced efficiency, reducing the need for manual labor, and decreasing errors in sorting and contamination during recycling.
Are there any examples of companies using AI for recycling?
Several companies are leading the AI recycling space, such as AMP Robotics, ZenRobotics, and Waste Robotics—all utilizing AI technology to optimize recycling processes and speed up sorting and waste recovery.